4 Steps to a Winning Data Strategy Roadmap
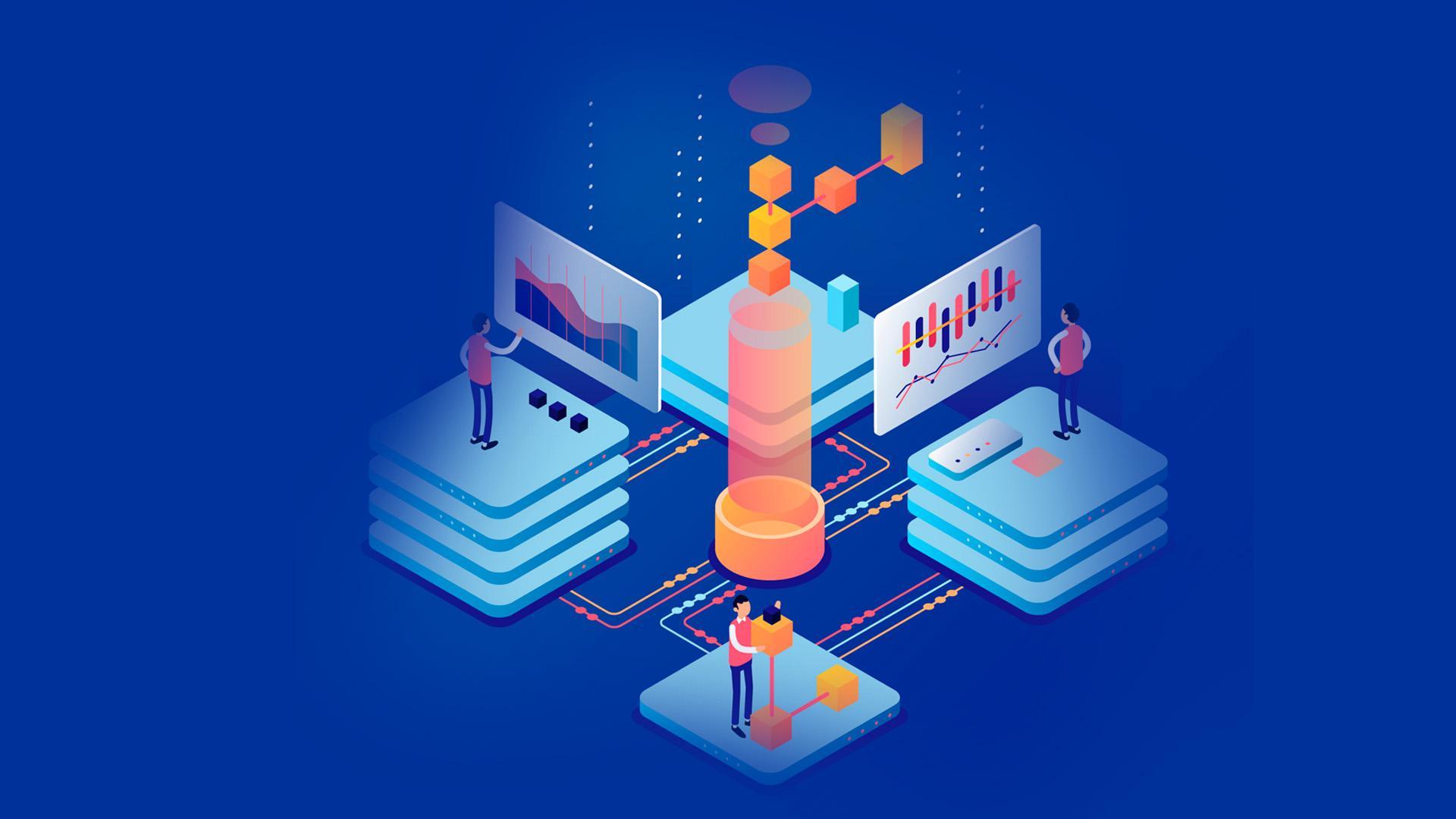
The ability to mine data and apply insights is a key factor in determining the leaders and laggards in any industry. Especially in Financial services Industry —where a huge volume of data remains unused— leveraging this ability becomes even more decisive to drive customer-centric products and experiences. To increase data access and enable more data insights, many firms are prioritizing modernization and reshaping their data architecture for a business-driven data strategy.
To embark on this journey, firms must address the challenges of a legacy system, including bad data quality, insufficient modeling, and poor alignment between people, processes, and digital tools. Designing a robust data roadmap that considers the organization’s business’s strengths, weaknesses, goals, and current technology stack is essential to build a data foundation for the future.
Based on OwlFinancial’s extensive experience in leading data modernization projects, below are four steps to help firms build a data strategy roadmap that will drive success to their data modernization journey.
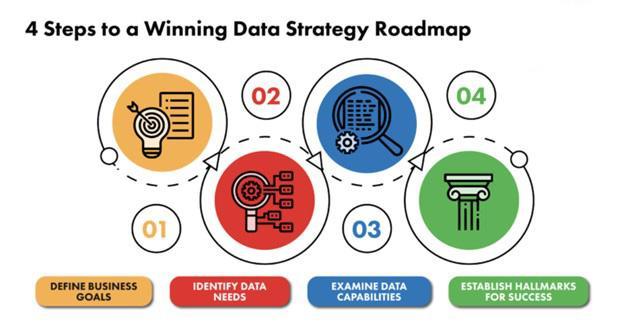
Step #1: Define Your Business Goals
Data projects, like any other projects, need to be aligned to the business goals of the organization. Before setting a strategy, firms should consider what the company wants to achieve. There are many use cases for data analytics in financial services, each of which can guide the data strategy. Some firms use cases include identifying risk, predictive analytics, uncovering fraud, marketing efficiency and customer experience.
By aligning the data strategy to specific use cases that are important to the business, measurable goals can be identified early on, and teams can establish signposts for the full data roadmap strategy and data governance implications.
Step #2: Identify Your Data Needs
Next, determine the major components required for the data architecture. These components fall into four classifications: data sources, data hub/data lake, analytics/BI, and business access. The basic definitions and considerations are outlined below:
Data Sources
- Internal structured data such as account holders name, address, and account number.
- Internal unstructured data like the narrative details within a loan.
- External structured data such as information such credit bereau or DMV information, for example.
- External unstructured data including insights from studies, news items, weather pattern reports, social media posts, or even drone photographs.
Data Hub/Data Lake
A data lake is the depository for sensitive and crucial information relevant to your business objectives, whereas a data hub refers to sharing that information across teams, platforms, and devices. Data lakes and hubs serve the critical function of consolidating structured and unstructured data to be tapped for insight. However, these repositories require tools to extract, reconcile, and transfer data from your legacy systems to the new data hub. They also need to integrate with the older systems (e.g., your core suite). To effectively use a data hub or data lake, you’ll have to consider where the hub will reside, such as the Amazon Web Services (AWS) cloud or Microsoft Azure.
Analytics/BI
Various tools and technologies utilize data hub to drive insights and data analysis. Since these hubs typically contain billions of data points, it’s impossible to mine, assess, and understand everything that’s available. Fortunately, applying AI-based analytics solutions can turn overwhelming quantities of raw data into usable insights.
Business Access
This is the interface layer for your business users. The easier these users can perform data analytics modeling, obtain reports, complete self-service tasks, and adhere to relevant data governance demands for privacy and security, the better they’ll extract value from the information you gather and organize. Tools that can help create simple dashboards or automate reporting should be evaluated.
Step #3: Examine Data Capabilities for Continuous ROI
Your data strategy roadmap brings every stakeholder together, and success shouldn’t end there. Instead, you must constantly refine your roadmap to account for fluctuating markets, new competitors, and changing customer preferences. To address new definitions of business value, it’s crucial to build three characteristics into the data strategy roadmap: modularity, automated and repeatable processes, and an effective support model.
Modularity
Organize your deliverables into smaller, logical capabilities so that various functions can work in unison with the same appropriate data sets. Then, utilize agile development and a DevOps approach to deploy capabilities swiftly and frequently with each module. This allows you to continuously iterate and harvest gains for the next cycle.
Automated and Repeatable Processes
Which tasks within your data strategy can be repeated? Standardize them to speed up development, rollout, and business utility. Given the size and scope of the data modernization ahead of you, it’s imperative to automate these processes wherever you can. Whether that’s processing loan application, sending and segmenting email campaigns, compiling underwriting information, sending account updates, performing compliance checks, or any other key task, find out how well you’re automating it and what the new technology stack can provide.
Effective Support Model
The people you rely on for effective data management, as well as the training required, must be a pillar of the roadmap. Consider how hiring and internal education might change as the demands of your modern data architecture evolve. At times, supporting your data initiatives may require partnering with a vendor for additional support.
Step #4: Establish Hallmarks for Success in Your Roadmap
To ensure the success of the data strategy roadmap, firms should build key pillars to support the execution of the strategy. These key pillars – or hallmarks for success – will enable firms to maximize value along the modernization journey. The key ones to establish are:
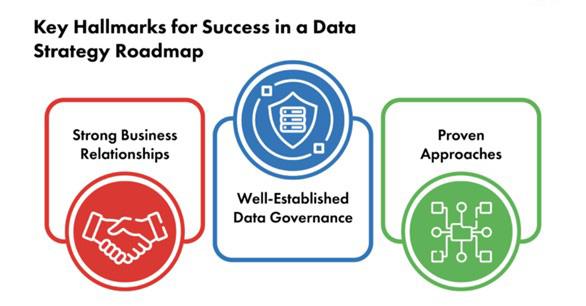
Strong Business Relationships
Strong relationships with key business stakeholders provide you with powerful champions for overcoming organizational inertia and keeping your data transformation initiative on track. Regularly delivering business-centric features and capabilities helps sustain enthusiasm.
Well-Established Data Governance
Having robust data governance is critical, especially with the volume of sensitive data in financial services firms. Establish clear policies and guardrails to help teams manage data appropriately and safely.
Proven Approaches
Leveraging proven architectures, frameworks, methodologies, and delivery processes not only reduce failure risk but also boost the likelihood of advantageous outcomes.
Achieving your Data Modernization Journey
Incorporating the aforementioned steps into your data strategy roadmap will set you up for modernization success and better business outcomes. With data-centric capabilities being a new imperative, financial firms who haven’t already made the move to modernize their data infrastructure will risk not only their bottom line but also their position in the industry.
Need more information? Leave a comment below and our team will get back to you shortly.